Machine Learning in Antibiotic Discovery
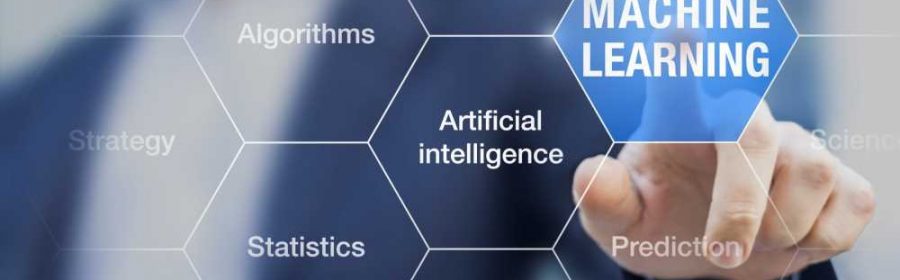
Machine learning in drug discovery
The current state of antibiotic discovery
Machine learning approaches in antibiotic discovery
Future outlook
References
Further reading
Machine learning is a significant component of artificial intelligence (AI), integrated into many fields, including data analytics and technology.
The drug discovery and development field has been accelerated with continuously evolving technology and computational science. However, with the popular emergence of AI and machine learning, this approach may have significant implications for drug discovery.
This article will provide insight into how machine learning, including antibiotic discovery, can be used for drug development.
Image Credit: NicoElNino/Shutterstock.com
Machine learning in drug discovery
Machine learning, which comprises algorithm-based techniques, depends on mathematical and computational theories that create models used in a wide variety of technologies including deep-learning assisted self-driving cars, advanced speech recognition, and more intelligent search engines.
These techniques were first explored in the 1950s and have since been used in versatile fields, including drug discovery. The high drug discovery and development expenditure has led to a healthcare burden, impacting pharmaceutical companies and patients requiring novel treatments.
The drug discovery process includes many steps, starting from identifying biological targets and characterization, leading discovery, optimization of lead candidates, and testing these compounds in vitro and in vivo before gaining Food and Drug Administration (FDA) approval.
This can be a lengthy process with a high rejection rate due to ineffective drug compounds that may not have the desired effect.
However, the use of computer-based approaches has advanced the field of drug discovery, being used for the discovery and optimization of lead drug compounds, such as through molecular docking, pharmacophore modeling, and decision forests.
The further application of computer modeling using novel machine learning algorithms can be useful for the entire drug discovery process rather than singular steps, which has the capacity to propel this otherwise lengthy process to have a higher success rate.
These algorithms have been used to develop models to predict drug compounds' biological, chemical, and physical characteristics. Other functions have included being used to find new drug applications, predicting drug-protein interactions, identifying drug efficacies, including safety biomarkers, and optimizing drug activity.
Examples of machine learning commonly used in drug discovery include but are not limited to Random Forest, Native Bayesian, and support vector machine.
The current state of antibiotic discovery
Interestingly, antibiotics have saved more human lives than any other type of drug over the years. However, there have only been a few new antibiotics developed over recent decades, with most being similar or only marginally different from already available drugs.
Additionally, the spread of antibiotic resistance has become a global concern, with many resistant populations being in danger of having ineffective treatment options.
The rising concern of antibiotic resistance at a global scale has required the adoption of novel approaches to discover new structural and functional classes of antibiotics that are still effective for resistant populations. Using algorithmic strategies to advance the antibiotic discovery process may be revolutionary for the global rise of antibiotic resistance.
While antibiotics are significant for patient health against bacterial infections, discovering novel antibacterial drugs has been a struggle for the medical and pharmaceutical fields.
Image Credit: iviewfinder/Shutterstock.com
Machine learning approaches in antibiotic discovery
The potential of contemporary machine learning and deep-learning models for high-quality datasets can be significant, with the possibility of forming predictions for novel antibiotics that may challenge the traditional laboratory screening process.
An alternative approach to overcome antibiotic discovery challenges can include using phenotypic drug discovery (PDD) screening strategies in order to select antimicrobial compounds for their capacity to prevent cell growth in vitro. The results of this screening method is dependent on the chemical diversity of the library that is used, with researchers using a combined multiparametric high content screening approach as well as PDD strategies and a data analysis pipeline that is supported by machine learning (Zoffman 2019). This approach enables novel identification of drug compounds, differentiating modes of action for successful lead compounds.
Additionally, researchers in MIT university have used a machine learning algorithm in order to identify a powerful novel antibiotic compound, which was found to eliminate many commonly problematic bacteria strains including some populations that are resistant to all well-known antibiotics.
This novel research utilized a computer model that has the capacity to screen more than a hundred million chemical compounds within days and works to select compounds that kill bacteria using a mechanism of action that is different to other available drugs.
The innovative model can also be used to design new drugs that is dependent on algorithmic learning about the type of chemical structure that aids drugs to effectively kill bacteria. It can also be used to optimize existing compounds through training the model to add characteristics to enable certain antibiotics to precisely target particular bacteria and prevent the elimination of good gut bacteria.
The researchers designed the model to identify chemical features that enable compounds to effectively kill E.coli, training the algorithm on 2,500 molecules, including 1,700 FDA-approved drugs and 800 natural products.
After being trained, the model was tested on a library of 6,000 compounds and predicted only one molecule to have strong activity against bacteria and low toxicity to human cells, which was also found to have a different chemical structure from existing antibiotics. The selected compound, named halicin, was not found to be resistant to E. coli during a 30-day period, and has been praised by researchers with the hope that it could be used within humans in the near future.
Future outlook
Machine learning has become a popular and powerful tool in today's technology and utilizing this in fields such as medicine and the antibiotic discovery and development may be revolutionary.
This can hold significant implications for the development of novel antibiotics, producing effective drugs that are more than just variants of existing drugs and instead carry a higher probability of success in eliminating bacteria strains without the risk of resistance.
References
- Anne Trafton, MIT News Office. Artificial intelligence yields new antibiotic. MIT News | Massachusetts Institute of Technology. Accessed July 12, 2023. https://news.mit.edu/2020/artificial-intelligence-identifies-new-antibiotic-0220.
- Patel L, Shukla T, Huang X, Ussery DW, Wang S. Machine learning methods in drug discovery. Molecules. 2020;25(22):5277. doi:10.3390/molecules25225277
- Zoffmann S, Vercruysse M, Benmansour F, et al. Machine learning-powered antibiotics phenotypic drug discovery. Scientific Reports. 2019;9(1). doi:10.1038/s41598-019-39387-9
Further reading
- Machine Learning for Disease Treatment
- AI and DL in Medicine
- Real time data in healthcare
Last Updated: Jul 18, 2023
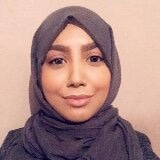
Written by
Marzia Khan
Marzia Khan is a lover of scientific research and innovation. She immerses herself in literature and novel therapeutics which she does through her position on the Royal Free Ethical Review Board. Marzia has a MSc in Nanotechnology and Regenerative Medicine as well as a BSc in Biomedical Sciences. She is currently working in the NHS and is engaging in a scientific innovation program.